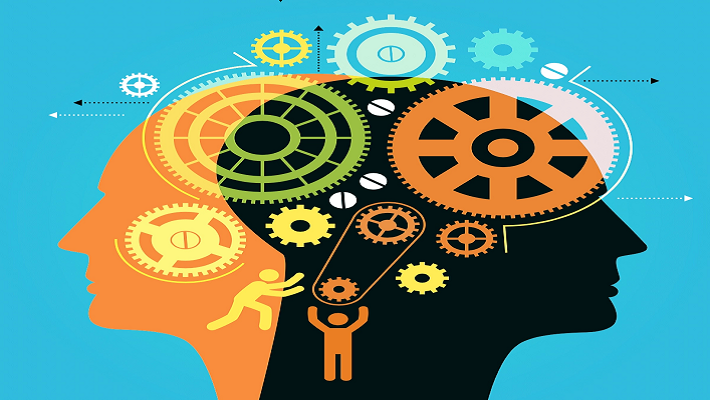
Buzzwords like digitization, big data and artificial intelligence are on everyone’s lips, and many companies and organizations have recognized the value of machine or deep learning for forecasting uncertain outcomes such as sales, production, or customer churn. However, when it comes to decision making about appropriately designing specific business activities like marketing campaigns or pricing policies, knowledge about the causal effects of those activities is required - something conventional machine-learning-based forecasts cannot deliver. This shortcoming has recently been overcome by the rise of causal AI, a modified version of Artificial Intelligence that is suitable for assessing the causal effects of business activities.
Utilising Causal AI for enterprises is the key to a revolution in machine learning. Causality is one of the key missing ingredients that’s needed to unlock real progress in AI. This is fast becoming the consensus view, within academia and industry. That being said, in this article let's look into the key points where causal AI will play a significant role.
Transparent Decision Making
First, causal AI is accountable for its acts and transparent in how it makes decisions. Decision-making processes are explicable and simple for humans to comprehend. This is due to the AI's deterministic methodology, which is built on a causal network that continuously learns and updates. This illustrates the usual safety engineering method of step-by-step fault tree analysis.
For instance, if the performance of an application declines, causal AI will examine dependencies to find abnormalities along the whole service delivery chain. It is able to pinpoint the particular cause of these. Additionally, it might reveal the problem's context and dependencies, highlighting the need for resolution-related action. This fault tree may be used by human operators to trace the AI's reasoning path and understand how and why it came to a particular conclusion.
Results can be reproduced
The capacity to replicate results is a fundamental aspect of science. The reproducibility and precision of scientific findings are improved when tests can be repeated and yield the same results. The same holds true for AI and computerised decision-making. Users must be aware of the great degree of repeatability of AI choices in order to trust them.
Owing to its transparent, comprehensively comprehensible, step-by-step fault tree analysis, causal AI enables reproducibility. Human operators may be certain that the conclusion was the right one and that it would be the same if a similar set of circumstances existed again since they can see the entire set of factors that went into the choice that was made. In the end, this raises confidence in the AI's judgments.
Elimination of Persistent Bias
Causal AI aids in removing decision-making bias that has been learned. Other types of AI, like machine learning, correlate data based on past data, but they do it in a mysterious way. Because AI choices are just the result of statistical convergence, frequently its creators are unable to describe how the AI makes decisions. These systems run the danger of bias by misreading signals or drawing the incorrect conclusion if they are fed inaccurate information or statistically converge with a bias.
Organizations need to give more attention to how to use AI in a way that goes beyond the time-based correlation of small data sets. In order for AI to identify and rule out causal relationships, it needs the whole context of the data that forms the basis of its conclusions. For instance, just because someone raises an umbrella doesn't mean it will rain. it would be incorrect for AI to draw the conclusion that rain and an umbrella go together. Because causal AI is aware of the relationships and interdependencies across IT systems, it can eliminate bias in AIOps.
Future of Causal AI
Causal AI is a fast developing field. Researchers are using it in areas as various as climate change and health, proving its vast potential as it becomes more evident.
A strictly data-driven strategy, however, is insufficient to address all development issues. To ensure that researchers and software developers understand causal networks accurately, expert knowledge must be incorporated at every stage of the process. By imposing limitations that reflect their understanding of how real-world systems operate and by determining if known confounding variables are absent from the data, experts may enhance the performance of causal AI.